Context Windows: How Developers and Artificial Intelligence Benefit from Developer Platforms
Context matters for both developers and artificial intelligence and they can both leverage a developer platform to improve contextual awareness
Developer ExperienceDeveloper PlatformAI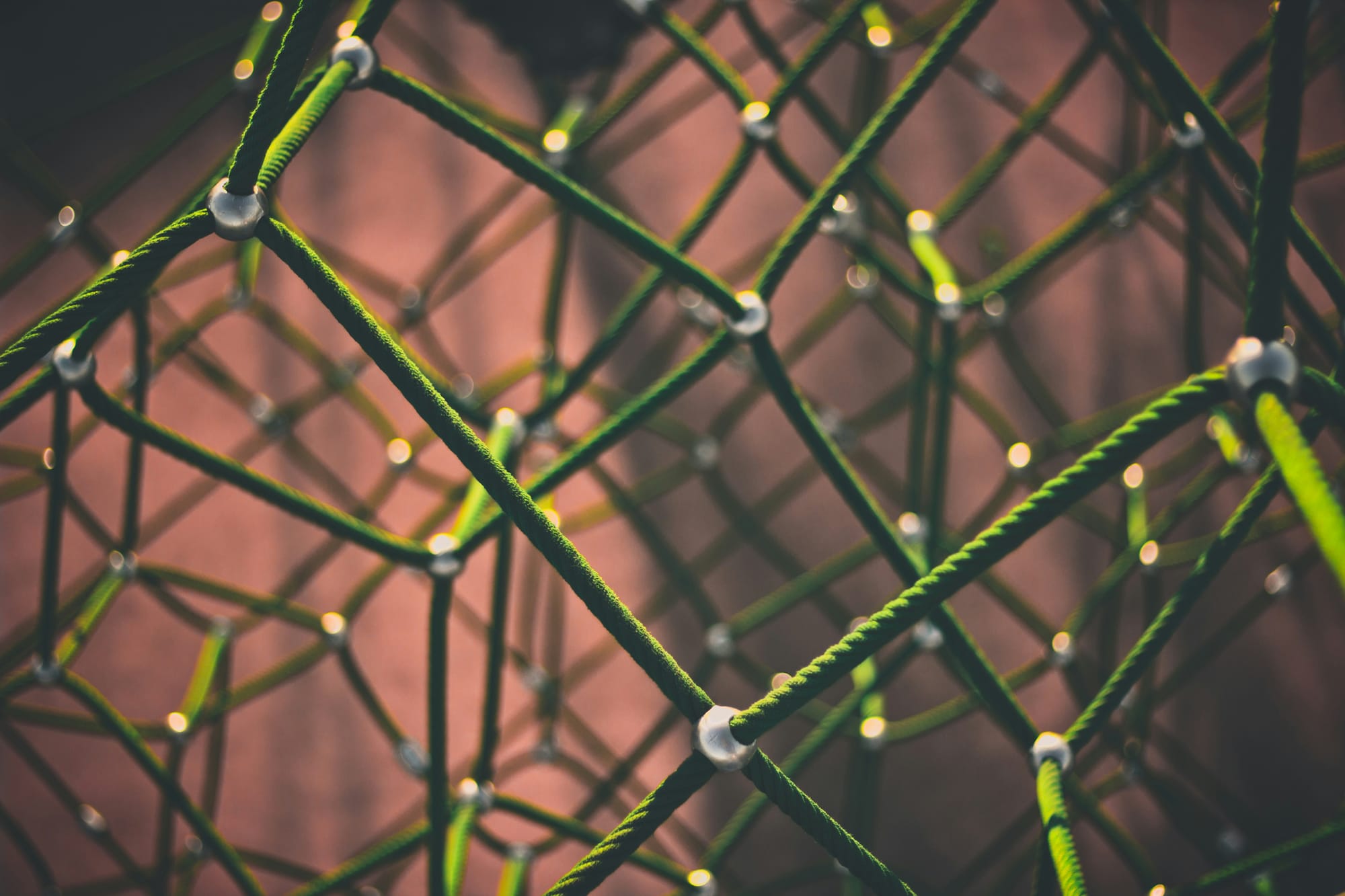
Why Context Windows Matter for LLMs and Developers
Context is critical for understanding and productivity for all roles but in particular for both large language models (LLM) and developers. For LLMs, the context window defines the amount of information the model can process at one time. Similarly, developers often deal with their own version of a "context window" as they juggle multiple tools, tabs, and technologies. By understanding how context windows work for LLMs and how developers can benefit from better context management, we can explore how developer platforms, agentic systems, and platform engineering streamline workflows and enhance productivity.
(Yes, we did just put the peanut butter, jelly and bananas into a sandwich.)
The Role of Context Windows in LLMs
Large language models operate within defined context windows, which set a limit on how much text or information they can "remember" during a given session. If the context window is too small, the model will lose important details, forcing users to repeat or reframe queries. With larger context windows, LLMs can:
- Process Complex Data: By analyzing more extensive data sets, documentation, or user requirements in a single interaction it can produce more comprehensive and accurate answers.
- Understand Nuance: Larger context enables better retention of conversational history, leading to more accurate and nuanced responses.
- Reduce Redundancy: Less need to reintroduce concepts or reframe questions.
However, even with large context windows, there are trade-offs. Increasing the context size can lead to slower response times and higher computational costs. Balancing these constraints is crucial for practical applications.
Developers and Context Switching
Developers frequently engage in "context switching" as they move between tools, browser tabs, and projects. Whether debugging an application, writing new features, or reviewing pull requests, a developers mental context window often becomes fragmented. This has significant downsides:
- Loss of Focus: Switching between tasks or tools disrupts concentration.
- Increased Cognitive Load: Keeping track of disparate pieces of information can be overwhelming.
- Opportunity Costs: Constantly reorienting slows down progress.
For example, imagine debugging a web application. A developer or might switch between an IDE, observability tools, database clients, browser-based tools, internal or external documentation, GitHub and maybe even a Slack thread. Each switch imposes a cognitive penalty, making it harder to build and maintain a mental model a given problem.
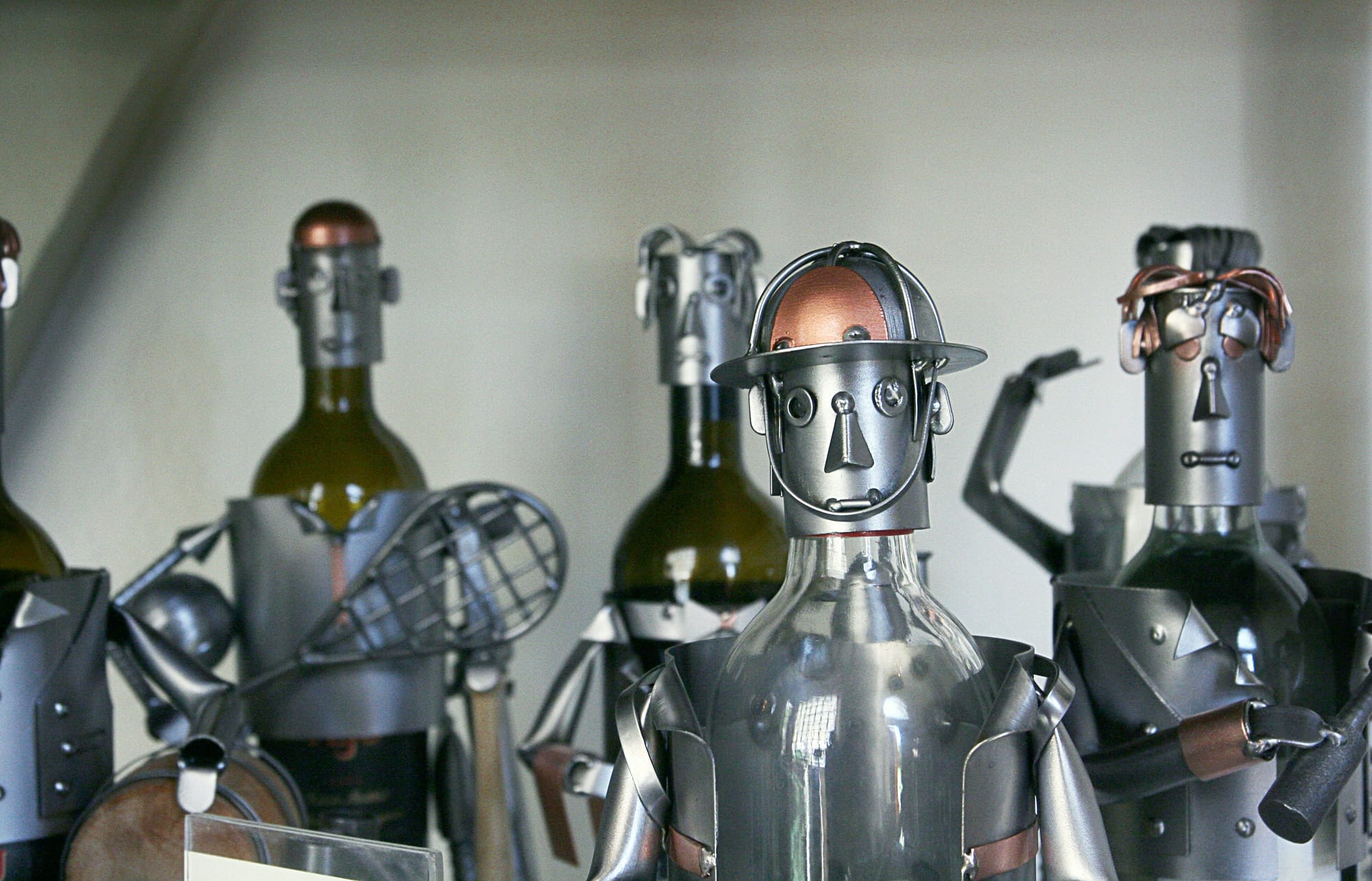
Agentic Systems and Networks of Agents: A Solution for Context Consolidation
Agentic systems—effectively networks of related tools working collaboratively—actively consolidate and manage context across various workflows. They deal with ongoing tasks and/or abstract tasks. Compared to so-called "one shot" LLMs there is a notable difference. By integrating these systems, developers benefit from enhanced productivity, reduced cognitive load, and better decision-making capabilities. Here’s a deeper look at how they function and the advantages they offer:
How Agentic Systems Work
Agentic systems operate by employing interconnected LLMs or specialized agents, each optimized for a specific task, data set or problem domain. These agents work collaboratively to:
- Integrate Diverse Data Sources: Agents collect and unify data from multiple tools such as APIs, SDKs, databases, version control systems, observability platforms, and communication channels. This ensures all relevant information is readily accessible.
- Maintain Workflow Context: By tracking developer interactions and workflows, these systems preserve context over time, enabling smooth transitions between tasks and reducing the need to reintroduce information.
- Dynamic Task Delegation: Each agent in the network specializes in a particular domain, such as analyzing logs, generating documentation, or debugging code. Tasks are dynamically assigned to the most relevant agent, optimizing efficiency.
- Feedback Loops: Agents continuously learn and refine their outputs based on user interactions and feedback, improving over time.
Networks of Agents in Practice
Networks of agents provide even greater functionality by enabling collaboration between individual systems. This requires comparatively smaller context windows when compared to a single "do it all" LLM. For example:
- Cross-Platform Integration: An agent monitoring a CI/CD pipeline can collaborate with another agent analyzing code repositories to pinpoint the root cause of a build failure.
- Enhanced Query Handling: One agent can summarize complex data from logs, while another provides relevant API documentation, delivering a holistic response to developer queries.
- Scenario Modeling: Networks of agents can simulate and evaluate various implementation strategies, offering recommendations tailored to project goals and iterating to improve based on feedback or results.
How Agentic Systems and Platforms Benefit Developers
- Reduced Cognitive Overhead: By automating the retrieval and synthesis of scattered information, developers can focus on higher-order problem-solving rather than mundane or repetitive tasks.
- Faster Debugging: With agents gathering relevant logs, traces, and documentation, developers can quickly identify and address issues.
- Improved Collaboration: Agentic systems can serve as intermediaries between team members or departments, summarizing discussions, highlighting dependencies, and tracking action items.
- Continuous Assistance: By proactively surfacing relevant insights during coding, testing, or reviewing, these systems keep developers in a state of flow.
Real-World Example: Enhanced Developer Platforms
We are a bit biased here, but let's consider a Backstage-based internal developer platform equipped with agentic capabilities:
- Automated Context Assembly: A well designed developer platform consolidates data from repositories and downstream developer tooling (logs, observability, CI/CD tools and even production environments) via integrations or plugins, documentation and issues into a single unified graph and interface.
- Task Prioritization: It highlights current state, surfacing relevant information into a "heads up display" for a given persona.
- Knowledge Sharing and Relevance: Unlike wiki's, spreadsheets and prior generations tooling current approaches seek to bring near real time data from downstream systems to the fore of developer workflows. This improves a developers situational awareness based on the information at hand, reducing the amount of time spent context switching and frankly working on "figuring it out".
By leveraging a system of this type a developer can achieve greater focus, faster results, and improved collaboration across teams.
Conclusion
It is worth highlighting that a normal developer has a smaller context window than an LLM, but human minds work differently than an LLM. Humans have a lower context switching cost and "recall cost" when remembering non-fresh information. Compared to current LLMs, you essentially need a new session or multi-agent network to approach the same levels of practical human performance.
What this really means is, that for certain situations, a human will produce better output faster when armed with the right tools. An LLM will thrive when a large initial dataset is fed into a context window. This is why we see LLM's thriving when it comes to junior and, maybe even, mid-level engineering work, but being less effective when it comes to advanced concepts.
Augmenting software teams with an agentic platform can further streamline all that goes into bringing code to production. Agents, when integrated well, help reduce context switching; allowing developers to work more efficiently and with greater clarity.
For both LLMs and developers, context windows define the boundaries of what’s possible.